O.2.16 - Machine Learning and agent based modeling for physical activity and nutrition research
Friday, May 20, 2022 |
15:10 - 16:40 |
Room 155 |
Speaker
An artificial intelligence approach to conducting pedestrian streetscape audits for physical activity
Abstract
Purpose: To train, test, and validate an artificial intelligence approach to detect micro-scale pedestrian streetscape amenities that promote walking and active travel. This work innovates by combining machine learning and computer vision techniques with Google Street View (GSV) images to overcome impediments to conducting streetscape audits, namely, the time and cost of expert human labor.
Methods: Step 1: We built deep learning classifiers using EfficientNETB5 architecture and Fast.ai deep learning framework for eight micro-scale features guided by the Microscale Audit of Pedestrian Streetscapes-Mini tool: sidewalks, sidewalk buffers, curb cuts, zebra crosswalks, line crosswalks, walk signals, bike symbols, and streetlights. Classifier output was a probability of feature presence in each image. Classifiers were developed using a train-correct loop, whereby classifiers were trained on a training dataset of images from of five U.S. cities, evaluated using a separate validation dataset, and trained further until acceptable performance metrics were achieved. Precision (i.e., positive predictive value), recall (i.e., sensitivity), and accuracy are presented. Step 2: Trained classifiers were used to conduct automated virtual audits for the home neighborhoods of participants in the Phoenix region, Arizona, USA enrolled in the WalkIT Arizona trial (N=512). Audit points included all coordinates for which GSV images were available within a 500-meter street network buffer around each participant’s home. We further explored correlations between model-detected micro-scale features and GIS-measured and participants’ perceived neighborhood walkability.
Results/Findings: Step 1: Classifier precision ranged from 100% (zebra crosswalks) to 87% (streetlights), recall ranged from 97% (walk signals) to 86% (sidewalk buffers), and accuracy ranged from 100% (zebra crosswalks) to 88% (streetlights). Step 2: The prevalence of model-detected features ranged from 90% (sidewalks) to 0.3% (zebra crosswalks). An index of model-detected micro-scale features was associated with GIS-measured macro-walkability (r=.30, p<.001). Positive associations were found between model-detected and perceived neighborhood sidewalks (r=.41, p<.001) and sidewalk buffers (r=.26, p<.001).
Conclusions: Automated virtual streetscape audits may provide a scalable alternative to human audits, thus enabling advancements in the field currently constrained by time and cost. Reducing reliance on trained auditors will enable scaling-up audits to assess hundreds or thousands of neighborhoods for population surveillance or hypothesis testing.
Use of deep learning and Google Street View imagery to examine relationships between residential streetscapes and physical activity.
Abstract
Purpose: Access to green space and walkable built environments affect daily routines and behaviors. Compared to satellite measures that provide a birds-eye view, Google Street View (GSV) images capture neighborhood microenvironments as viewed and experienced by residents. Here we examine relationships between residential GSV-derived built environment features and physical activity (PA) within the Washington State Twin Registry (WSTR).
Methods: We examined 9,483 adult twins enrolled in the WSTR from 2009-2019 living in urban areas; 16,701 total survey observations were analyzed. The PSPNET deep learning segmentation algorithm was applied to images sampled from 100 meters around residential addresses to quantify street level objects. We created exposure metrics hypothesized to be important to PA, including %total green space, %accessibility features (sidewalks, paths, stairs, streetlights, benches, step, etc.), %built environment features, %sidewalks separately. Bouts and duration of PA, including moderate-to-vigorous PA (MVPA) and neighborhood walking, were self-reported. Those with ≥150minutes/week of MVPA and walking were coded as meeting recommendations. Mixed effects logistic regression models determined odds ratios (ORs) of meeting recommendations for MVPA and for each quartile increase in residential GSV measures. Models included a nest random intercept for twins and twin pairs, and adjusted for age, sex, race, income, neighborhood area deprivation, and satellite-derived greenness (NDVI within 100m).
Results/findings: Across observations, most WSTR participants did not meet PA recommendations for MVPA (62.1%) or walking (76.2%). Positive relationships between MVPA and increased GSV measures were observed, however were not significant in fully adjusted models. Specifically, the OR for meeting MVPA recommendations was 1.10 (95%CI: 0.96-1.26) for total greenspace, 1.09 (0.96-1.24) for accessibility features, 0.97 (0.85-1.11) for built environment features, and 1.12 (0.98-1.27) for sidewalks, all comparing the top quartile of exposures to the lowest. For walking we observed similar associations; the OR for meeting recommendations was 1.16 (0.96-1.39) for total greenspace, 0.99 (0.84-1.17) for accessibility features, 0.92 (0.77-1.10) for built environment features, and 1.10 (0.92-1.30) for sidewalks, comparing the top quartile of exposures to the lowest.
Conclusions: We observed small associations between street-level GSV measures and PA. Further research will integrate satellite and street-level measures to capture built environment characteristics important to PA.
Examining the impact of large-scale built and food environmental changes on physical activity and healthy eating using agent-based modeling
Abstract
Purpose: Testing the impact of systems-level changes to the built and/or food environment using traditional controlled experimental designs is not always feasible. This study aimed to demonstrate the utility of agent-based modeling for assessing the potential effects of large-scale modifications to the built and food environments on physical activity and healthy eating outcomes.
Methods: We developed two independent agent-based models. The first simulates three city types (low-, middle-, and sprawling high-income country cities), and tests the impact of five scenarios (vs. business-as-usual) on physical activity and sustainability outcomes. Scenarios were: 1) expansion of public transit infrastructure; 2) expansion of pedestrian and bicycling infrastructure; 3) expansion of public open spaces; 4) strategies 1-3 combined; 5) strategies 1-3 combined, plus increased driving costs. The second model simulates the City of Austin, Texas, USA, and tests the impact of four food environment change scenarios on vegetable consumption among low-income, ethnically-diverse residents. Scenarios were: 1) expansion of non-traditional food assets in low-income communities (farm-stands, mobile markets, healthy corner stores); 2) reduced cost of vegetables in existing non-traditional food assets; 3) expansion of non-traditional food assets plus 50% cost reduction for vegetables; 4) cost reduction for vegetables in traditional food assets (supermarkets/grocery stores).
Results: For the built environment and physical activity/sustainability model, the “all strategies and no increased driving cost” scenario was the most optimal one for improving population levels of physical activity and sustainability outcomes (improved air quality, decreased carbon emissions, reduced traffic deaths). In the sprawling, high-income country city type, only the “all strategies plus increased driving costs” scenario showed meaningful population-level improvements in physical activity and sustainability outcomes. The food environment model showed that steep reductions (>70%) in vegetable prices in supermarkets/grocers is a as effective a strategy for increasing vegetable intake among low-income residents, as is increasing the number of non-traditional food stores (mobile markets) whilst offering a 50% discount on vegetables.
Conclusions: Large-scale modifications to the built and food environment of cities can be effective strategies for promoting physical activity and healthy eating, and for reducing health disparities. Multi-component, synergistic approaches help maximize public health impact and minimize unintended consequences.
Development and face validity testing of MealSim, an agent-based model simulating child eating behaviors
Abstract
Purpose:
The purpose of this study is to develop an agent-based simulation model of the school meal environment that can be used by school nutrition administrators and policymakers to identify evidence-based strategies to improve child diet quality and reduce wasted food in school meal programs.
We developed the agent-based model in three stages. First, we review the relevant literature to build a conceptual model of child eating behaviors during the National School Lunch Program and identify key variables associated with the school meal environment to include in the model. Second, we apply econometric models to quantitative data from cafeteria experiments to predict student food selection and consumption. Third, we use these findings to develop the agent-based model in the NetLogo programming language. The model simulates a complex system consisting of a school cafeteria with food, meal policies, and heterogenous students applying the econometric models and other boundedly-rational strategies to make decisions about food selection and consumption. Our multidisciplinary team conducted verification processes to ensure the agent-based model reflects underlying economic and behavior theory and other relevant systems factors. Face validity testing was conducted with school nutrition staff, relevant community partners, and academicians.
Results:
Face validity testing suggests that the model has relevant applications to the work and interests of school nutrition practitioners and their relevant community partners, as well as use in future research on the impact of new school meal policies. Constructive criticisms included the need for improved integration of peer effects and agent-to-environment interactions.
Conclusions:
The base MealSim model adequately reflects the current evidence of child eating behaviors during lunch and is well-received by practitioners and researchers. Additional research is needed to incorporate face validity findings and validate the model.
FLASH-TV 2.0: Refining and assessing the FLASH-TV methods for TV viewing estimation
Abstract
Purpose: Excessive TV-viewing among children is a public health concern, yet tools to measure children’s TV viewing suffer from biases. Our goal was to refine and reassess FLASH-TV 1.0, an objective measure of children’s TV viewing using computer vision and machine learning algorithms to analyze video images of children in front of TVs.
Methods: Four design studies (n=21) were conducted with family triads (parent and 2 siblings): three in an observation lab and 1 in the child’s home. Family triads participated in task-based screen use protocols for about 90 minutes. The FLASH-TV system included a video camera placed near TV facing the room in front of TV during data collection. Video data coded by staff using duration coding for whether the target child’s gaze was on the TV were the gold-standard (10% double coded, mean Kappa 0.83-0.88). FLASH-TV estimated a child’s TV viewing time by sequentially detecting faces in a video frame, verifying that the face was the target child, and assessing TV-watching (gaze) behavior. Enhancements of convolutional neural network algorithms for each step included substituting YOLOv2 for RetinaFace for face-detection; DeepFace for ArcFace for face verification; and using a combination of Gaze360 and ETH-XGaze for gaze estimation. Additionally, the video-data were assessed at 5-second epochs to reduce the noise in the system. The target child’s TV viewing duration estimated by FLASH-TV running the three steps sequentially was compared to the gold standard, with criterion validity for overall TV viewing calculated using intra-class correlation (ICC) in a generalized linear mixed model.
Results: The children’s mean age was 10.2 years, with 38.1% non-Hispanic white, 28.6% black, 19% Hispanic white, and 14.4% other. Face detector’s overall sensitivity improved from 93.6% to 96.1%. Face verification overall positive predictive value improved from 90% to 96% reducing the false positive rate. The ICC improved from 0.725 to 0.961 when comparing the child’s gold standard TV viewing time (minutes) to FLASH-TV estimated time.
Conclusion: FLASH-TV 2.0 significantly improved the performance of FLASH-TV 1.0 to identify when a target child is watching TV and offers a critical new tool to accurately measure children’s TV viewing.
Nutrient Prediction on Recipes – Use Case on Heterogeneous Recipe Datasets
Abstract
Objective: Nutrient tracking is the process of breaking down nutrient ratio while tracking meals to ensure eating according to a certain dietary goal. There are plenty of apps for tracking that help users in this, as well as recipe datasets – a valuable source of information for meal planning and grocery shopping. Experts in numerous industries are continually looking for ways to improve and simplify the process of estimating nutritional values that are missing for different reasons. The aim of this study was to explore the performance of a machine learning pipeline for predicting nutrient values from text embeddings on heterogeneous recipe datasets (i.e., datasets that come from different sources) for benchmarking.
Methods: Our proposed machine learning pipeline predicts the nutrient profile of a recipe by using text embeddings on the ingredients, combined with a domain heuristic. The domain-specific heuristic merges the embeddings of the ingredients by incorporating their quantities. We evaluated the pipeline by using seven heterogenous recipe datasets. One of the datasets, called Spoonacular has nutrient values available, while all the others have no nutritional information. To apply the methodology, before the predictions, the datasets went through the processes of named entity recognition (for extracting the measurement units and quantities of the ingredients), and data mapping (for mapping the ingredients to a Food Composition Database (USDA) to obtain the nutrient values).
Results: The prediction models were highly effective for all seven datasets. Regarding the separate nutrient predictions, the best results were achieved from the protein prediction models (up to 95% accuracy) and the worst performing were the salt prediction models (up to 51%). On a dataset level, the best results were achieved for the Spoonacular dataset, while the accuracies for the other datasets were approximately 12-16% lower in accuracy.
Conclusions: With this use case we achieved harmonization over the meta-data of different heterogeneous recipe datasets, while evaluating the effectiveness of the machine learning pipeline and the domain heuristic for merging multi-word embeddings. The diversity of the data in these datasets also provides a base for generalizing the prediction models to many different scenarios with minimum amount of data.
Co-chair
Session Chair
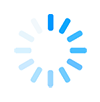