O.2.21 - Environmental measures for physical activity and nutrition
Friday, June 19, 2020 |
11:15 AM - 12:45 PM |
Waihorotiu #1 Level 4 |
Details
Speaker
Developing automated methods to collect, store and characterise by cuisine type sold, large volumes of fast-food outlet data: a feasibility study.
Abstract
Purpose: Research investigating associations between physical exposure to fast-food outlets and dietary behaviour is commonly cross-sectional, limiting causal inference. Moreover, despite variation in nutrient profiles of food sold, associations have not been tested by fast-food outlet cuisine type (e.g. burgers, fried chicken, pizza). These knowledge gaps could be addressed using well-characterised, longitudinal data. The Food Standards Agency (FSA) now publishes real-time data on all UK fast-food outlets (n=>60,000) via an Application Programming Interface (API). These data have significant research potential, but are not available retrospectively, and outlets are not characterised by cuisine type sold. Repeated manual data collection and storage, and characterisation of outlets by cuisine at this scale, is unrealistic. We test the feasibility of using innovative data science methods to automate data collection, storage and prediction of outlet cuisine type from business name alone.
Methods: We wrote a python script to collect outlet data weekly from the API, for storage in a custom database. To characterise outlets by cuisine type we developed a model using machine (deep) learning. The model used a ‘training’ dataset from another provider, of 15,000 fast-food outlets, where each had been labelled as one of nine major cuisine types, to learn the ‘language’ of how outlets of different cuisines are named. We tested the accuracy of our predictions on a separate dataset of 5,000 outlets.
Results: Data were collected automatically 22 times between September and November 2019. Overall, our model had 65% cuisine type prediction accuracy. Prediction rates varied by cuisine type (in descending order): Fish & Chips (81%), Chinese (77%), Desserts (77%), Indian (76%), Chicken (74%), Pizza (62%), Bakery (61%), Kebab (56%), Burgers (40%).
Conclusions: It was feasible to automate weekly collection and storage of FSA data. This longitudinal dataset is unprecedented and will be made publicly available to underpin public health research. Early machine learning results are promising, but indicate imperfect cuisine type prediction. We will refine our model to improve accuracy, and liaise with end-users to maximise accuracy for priority cuisine types. Our final model will be applied to stored FSA data, and could be adapted for application to international data.
Investigating associations between child body size and exposure to the nutrition environment: considering the home and school neighbourhoods and routes to school
Abstract
Purpose: For over a decade, there has been considerable research linking the nutrition environment (NE) and children’s body size. However the evidence is mixed and objective methods to measure associations widely differ. The aim of this study was to better quantify children’s exposure to the NE around the home, school and along the route to school, using novel geospatial techniques, and test for associations with children’s body size.
Methods: Data from the Neighbourhoods for Active Kids (NfAK) study was used to quantify children’s exposure to the NE. NfAK is a cross-sectional study of 1102 children aged 8-13 years across 19 schools in Auckland, New Zealand. Children’s waist-to-height ratio was the primary outcome of interest. The NE was categorised according to ‘unhealthy’ (e.g. fast food outlets) and all food types. For every child, three exposures to the NE were created around the 1) home, 2) school and 3) along the route to school (child-drawn). A number of Geographical Information System (GIS) techniques, including kernel density estimation and network analysis, were used to develop child-specific exposure measures around the home and school. Multilevel regression analysis was used to examine associations between NE and children’s body size for each of the neighbourhood exposure types. Models were adjusted for age, sex, ethnicity, neighbourhood deprivation, travel mode and whether children were accompanied or not by a parent on their way to school.
Results: Preliminary results indicate that in all three environments, high exposure to ‘unhealthy’ food environments were associated with increased body size in children. Mode of travel to school and whether children were accompanied or not by a parent to school also affected their exposure to ‘unhealthy’ food in the NE. Further analyses are currently being undertaken and will be presented and discussed in this paper.
Conclusions: This study used a novel child-centred approach to quantifying the exposure of the home, school and route to school environments and testing associations with children’s body size. More specific and refined geospatial measurement approaches are necessary to improve the evidence base necessary for influencing public health policies and improving child health outcomes.
Is it Physical Activity or Green Space that Impacts Adolescent’s Mood? – An Ambulatory Assessment Study.
Abstract
Purpose: Contextual factors have a critical impact on human behavior and mental health, with ambulatory assessment as the state-of-the-art approach to collect data on all three components: Through combining accelerometer-measured physical activity (PA), e-diaries on smartphones to assess mood in real-time, and geolocation-tracking, ambulatory assessment allows to investigate within-person processes in human's everyday life.
The within-person relationship between PA and mood has been investigated in several ambulatory assessment studies, but results are inconsistent. This might be due to a neglect of contextual factors. Thus, we tested if PA and green space (GS), a well-researched contextual factor, show distinct effects or confound each other in predicting mood.
Methods: Within the URGENCY study (https://www.zi-mannheim.de/en/research/research-associations/pez.html), adolescents (N = 141, 42.5% female, mean age = 15.07; BMI = 20.4) wore accelerometers for seven consecutive days. Additionally, they responded on average six times per day to mood-questionnaires on GPS-triggered smartphone-diaries during everyday life. We calculated the percentage of GS within 100m2 around the participants geolocation (using ArcGIS), parameterized GS and PA across different time frames prior to the e-diary prompts, and applied multilevel model analyses (using SPSS) to test for within-subject effects of PA and GS on mood.
Results: If using separate models for PA or GS predicting mood dimensions, both PA and GS were positively associated with affective valence (both p <. 01) and energetic arousal (both p < .05), but calmness was only negatively impacted by PA (p < .05). However, entering both PA and GS simultaneously into the models changed the results for affective valence, which was only associated with GS (p < .05). No interaction effects were found between PA and GS.
Conclusion: PA and GS show distinct effects on adolescents’ mood in everyday life: Only GS enhances affective valence, both GS and PA increase energetic arousal, but only PA decreases calmness. If replicated, these results can guide interventions using PA and GS to regulate adolescents’ mood. Moreover, they may explain inconsistent findings from within-person studies, suggesting that future studies should not only measure PA, but also include contextual factors.
Shall we go to the park and work out? A comparison of outdoor exercise equipment use in a Western Australia neighbourhood in 2012 with 2019
Abstract
Purpose: Parks are an important physical activity resource as they are accessible and provide a place to be active. Easy-to-use, park-based outdoor gyms were a novel opportunity embraced by local governments a decade ago. In 2012, two Western Australia (WA) parks installed weight-based park exercise equipment; in 2018 the equipment was replaced by resistance-based equipment. The aim of this study was to evaluate the use, and perception of outdoor gym equipment in two metropolitan WA parks and compare the changes over a seven year time period (2012 with 2019).
Method: A mixed methods design was used. Data were collected in 2012 and 2019 in the City of Belmont via observations of equipment users using a modified (SOPARC) tool, and intercept interviews with equipment users. Data were collected in summer and winter.
Results: In 2012 n= 833 and 2019 n=909 people were observed at the two parks. In 2012 6.6% of park visitors used the gym equipment compared with 8.6% in 2019. More males used the equipment at both parks in 2012 (66.7%); 2019 (71.8%). In 2012 (n=43) park users completed the intercept interviews and reported using the equipment 2-3 times per week, for 10 minutes or less. In 2019 n=63 interviews were completed. Of the interviewees 66.7% reported their physical activity level had increased since using equipment. In 2019 there was a significant increase in use of the outdoor gym equipment when comparing summers, and a significant decrease when comparing winters.
Conclusions: Park based gym equipment is a feasible method to promote low cost, physical activity opportunities however remains underutilised. The research confirmed few visiting the park used the gym equipment, and highlighted the need to explore strategies to increase equipment use including social support and clear guides on how to use the equipment. The findings provide local government planners and policy makers with data to support the use of local parks for low cost physical activity opportunities.
Assessing the school neighbourhood built environment: Modification of an environmental audit tool and protocol (MAPS Global-SN)
Abstract
Purpose
Active transport to school contributes towards adolescents’ daily physical activity and can be influenced by school neighbourhood built environment (SN-BE) characteristics. SN-BE assessment typically involves micro-scale (i.e., environmental audits) or macro-scale (Geographic Information Systems (GIS)) tools. However, existing environmental audits are time/resource-intensive and not specific to school neighbourhoods, while GIS databases are not generally purposed to include micro-scale data. This study evaluated the inter-rater reliability and feasibility of using a modified audit tool and protocol (Microscale Audit of Pedestrian Streetscapes Global–School Neighbourhood (MAPS Global-SN)) to assess the SN-BE of secondary schools. Correlations between MAPS Global-SN and GIS measures of the SN-BE were also examined.
Methods
MAPS Global-SN audit (adapted from the original MAPS Global audit) and GIS spatial analysis (intersection density, residential density, land use mix, walkability) was conducted within a 0.5 km street-network buffer-zone around all twelve secondary schools in Dunedin, New Zealand. Based on investigator and expert consultation, MAPS Global-SN included eight modifications to both auditing processes and items. Inter-rater reliability data was collected from two independent auditors across two schools. Intraclass correlation coefficient (ICC) was calculated for nine MAPS Global-SN overall sub-scales and scores. The feasibility of a condensed audit protocol (auditing one side of each street segment in the neighbourhood, compared to both sides) was also assessed. Data were analysed using Pearson’s Product Moment Correlations and Spearman's Rank Correlation Coefficient.
Results
Overall, all assessed sub-scales and scores showed "good" to "excellent" inter-rater reliability (ICCs=0.60-0.99). When considering the condensed audit protocol, significant positive correlations were identified between odd and even sides of each street segment for five of eight assessed sub-scales and scores (r=0.68 to r=0.98; all p<0.05). Only one of the overall MAPS Global-SN sub-scales was positively correlated with school-level GIS measures of intersection density, residential density and walkability (r=0.64 to r=0.75; all p<0.05).
Conclusions
MAPS Global-SN and condensed protocol may be a feasible alternative to micro-scale SN-BE assessment, by simplifying data collection and time/resource commitments. Macro-scale GIS analysis should complement MAPS Global-SN. Future studies should establish the reliability of MAPS Global-SN internationally and assess the SN-BE in relation to active transport to school.
Training computers to see the built environment for physical activity: automated detection of sidewalks using computer vision and Google Street View images
Abstract
Purpose: Sidewalks are vital built environment features to encourage safe walking and other physical acitvities, but data on the location or presence of sidewalks on US streets is often non-existent. Virtual audits of environmental features using Google Street View (GSV) are reliable alternatives to traditional in-person audits. However, because audits must be conducted by humans, virtual audits remain time-intensive and are susceptible to auditor fatigue. The scalability of virtual audits remains dependent on the amount of available trained human labor.
Objective: To train, test, and validate an artifical intellegence approach to detecting sidewalks in Phoenix, Arizona, USA.
Methods: Researchers labeled 557 GSV images (512x512 pixels) in Phoenix using semantic segmentation (i.e., each pixel classified as sidewalk vs. not sidewalk) to identify the presence and location of sidewalks for training of deep neural networks. An additional 601 images with existing segmented sidewalks labels from the Cambridge-driving Labeled Video Database (CAMVID) augmented this training dataset. Training data were used to develop deep-learning models for sidewalks, based on a Fast.ai deep-learning architecture (i.e., dynamic U-Net based ResNet-38). Researchers also developed an independent dataset of images to validate trained models (i.e., 200 GSV images from Phoenix and 101 from CAMVID). Model-detected sidewalk features were compared to human ‘ground-truth’ features during testing and validation using pixel-level comparisons with standard machine learning metrics: positive predictive values (PPV), negative predictive values (NPV), and sensitivity.
Results: Sidewalks were sufficiently present in both the training (96%) and validation (94%) datasets. During validation, if the computer model detected sidewalks, the probability that the pixel-level detection agreed with human raters (PPV) was 90%, with sensitivity values of 90%. For non-sidewalk pixels, the model agreed with humans 99% (NPV) of the time. Validation against Phoenix-only images suggested lower, but still acceptable validity (PPV=0.83, NPV=0.99, sensitivty=0.81).
Conclusions: Machine-learning approaches validly detected sidewalks in GSV images. Results confirmed visual inspections showing a close match between computer-inferred and human raters’ sidewalk locations. Next steps include further generalizability and construct validity testing across thousands of neighborhoods with participant physical activity levels.
Development of a Cycling Scale for Preschool Children
Abstract
Learning to cycle independently is a milestone for most children, one which requires time and practice to master. A measure of cycling ability would allow accurate assessment of the development of cycling and would provide teachers, practitioners and parents a valuable tool to further encourage uptake of cycling in early childhood. Cycling is an important skill to promote in early childhood and throughout life as it has many health benefits along with being a commonly reported physical activity globally. To date, there are no measurement tools examining the developmental process to independent cycling in early childhood. The current study aimed to develop a cycling scale and to further assess the development paths children took across a 5-week cycling intervention. Development of the scale occurred in four phases: (1) development of criteria and stages, (2) review of instructions and criteria and pilot inter-rater and test-retest reliability, (3) 5-week cycling intervention and (4) final inter-rater and test-retest reliability. Three hundred and forty-nine children between 2 and 6 years took part in one of the four phases. The developed scale included eight stages in total. The scale was found to have excellent inter-rater reliability (ICC = 0.97, 95% CI = 0.96-0.98) and good to excellent test-retest reliability [(ICC = 0.91, 95% CI = 0.87-0.94) & (ICC = 0.90, 95% CI = 0.85-0.93)]. Typical routes to independent cycling along the scale were observed and reported as being step-wise except one instance where a two-stage jump was as common as the step-wise route. Alternate routes were also observed and reported on. The current study developed a reliable measurement tool for assessing children between 2 and 6 years of age on the developmental process to independent cycling. The developed cycling scale will allow teachers, practitioners and parents to assess competence in cycling and moreover, track changes in skill development. Furthermore, the scale could also be used to better understand and better asses a child’s progression when learning to cycle.
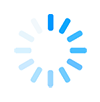