S.1.02 Novel accelerometer data processing methods for quantifying movement behaviors in free living environments
Thursday, June 18, 2020 |
8:30 AM - 9:45 AM |
Hunua #2 Level 1 |
Details
Speaker
Evaluation and comparison of laboratory-based and free-living activity recognition models for preschool-aged children under free-living conditions
Abstract
Machine learning activity recognition models provide researchers with alternative activity metrics in addition to intensity. However, existing algorithms for preschool children have been trained on data from laboratory-based activity trials and their performance has not been investigated under free-living conditions.
Purpose: To evaluate the accuracy of laboratory trained hip and wrist Random Forest (RF) classifiers for automatic recognition of five activity classes: sedentary, light household activities and games, moderate-to-vigorous sports and games, walking, and running in preschool children under free-living conditions. In addition, the performance of the laboratory trained models was benchmarked against models trained on free-living data.
Methods: 31 children (4.0 ± 0.9 yrs) were video recorded using a GoPro during a 20-minute unstructured active play session. Participants wore an ActiGraph GT3X+ on their right hip and non-dominant wrist. A bespoke two-stage direct observation scheme was used to continuously code ground truth activity class, and to identify which movement behaviours contributed to misclassification errors, the specific activity types occurring within each class.
Twenty-one of the children were randomly selected to train free-living RF classifiers for the hip and wrist. Performance of the laboratory and free-living classifiers was subsequently assessed in the hold-out ten children by calculating overall recognition accuracy, kappa statistics, and generating confusion matrices summarising class level accuracy.
Results: Accuracy for the hip and wrist laboratory trained RF classifiers was 67.5% (κ = 0.42) and 56.9% (κ = 0.32) respectively. In comparison, accuracy for the free-living trained hip and wrist RF classifiers was 83.1% (κ = 0.70) and 79.7% (κ = 0.64), respectively. The free-living RF classifiers provided substantial improvement for classification of sedentary (5.6% - 11.3%), light household activities and games (10.2% - 26.7%), walking (43.5% - 65.0%) and running (16.6% - 22.2%).
Conclusions: Laboratory trained activity recognition models for preschool aged children do not perform well when implemented in new data collected under true free-living conditions. In contrast, classifiers trained on free living data perform well. These findings support the view that machine learning activity recognition models be trained under free-living conditions.
Activity classification models for children: how well do lab-developed models generalise to free-living conditions?
Abstract
Purpose: Classification of activity behaviours using raw accelerometer data is becoming more prominent. Almost all activity classification algorithms are developed using data collected in controlled laboratory environments which may not be generalisable to free-living settings. This study examined how machine learning models trained on laboratory data performed in free-living settings, and how the accuracy changed when the models were retrained with additional free-living data.
Methods: In a lab setting 40 children (19 males, aged 10.1 ± 1.7 years) were equipped with two Axivity AX3 accelerometers worn on their thigh and lower back. They performed a series of activities (e.g., sitting, standing, walking, running, lying) that were captured by video camera (criterion measure). Fifteen new children (10 male, aged 10.0 ± 2.6) wore the same two accelerometers and a small wearable video camera that captured their free-living movement behaviours.
Using the lab dataset, a random forest was trained to classify each activity using several features of the accelerometer data (e.g. axis means). After this model was evaluated in the lab setting, it was used to predict activity type in the free-living dataset. As a last step, the model was retrained with both the lab and free-living data together, and the accuracy was estimated using leave-one-subject-out-cross validation.
Results: The accuracy of the lab-trained model was 97.8% (95% CI: 97.6–98), kappa κ = 0.98. This dropped to 92% (91.6–92.5), κ = 0.88, when applied to the free-living data. Retraining the model with additional free-living data improved the free-living accuracy to 97.2% (97–97.4), κ = 0.96.
Conclusions: Activity classification models developed in a laboratory setting showed a ~6% reduction in accuracy (10% reduction in κ) when applied in a free living setting. Accuracy improved when models were retrained with additional free-living data. Future studies should include free-living data when developing classification models to ensure their generalisability.
Can free-living activity classification models developed in healthy adults be used in a dialysis patient population?
Abstract
Purpose: Machine learned (ML) models developed to classify activity behaviours from raw accelerometer data in free-living were shown to be accurate (82.7%, kappa κ=0.74) and demonstrated epidemiological utility in over 90,000 UK Biobank participants. However, it is unknown how well these ML models generalise to inactive diseased populations. We therefore examined if ML models trained on free-living data from healthy adults could be used in a dialysis patient population.
Methods: 153 healthy UK adults were asked to wear an Axivity AX3 accelerometer on the dominant wrist for 24 hours and a Vicon Autographer wearable camera to capture their free-living movement behaviours. In a separate study, 25 adults with end-stage kidney disease on maintenance dialysis were asked to undergo the same accelerometer and wearable camera protocol. Camera data was labelled by trained annotators into four classes: sedentary behaviour, light tasks, moderate activity, and walking.
Random Forest models were trained to classify activity type from 132 time and frequency domain features for each 30 second epoch, with a Hidden Markov model used to smooth predictions. The models developed in healthy adults were then applied to data from dialysis patients. Models were then retrained using dialysis patients’ data only. Finally, models were retrained with a mix of healthy and dialysis patient data together.
Results: When the model trained in healthy adults was applied to dialysis patients, accuracy was 74.3% (κ=0.16). When trained in dialysis patients only, models achieved accuracy of 72.5% (κ=0.19). When trained in healthy adults and dialysis patients, models achieved accuracy of 74.4% (κ=0.15) on dialysis patients only.
Conclusions: Activity classification models developed in a healthy population achieved substantially lower accuracy and kappa statistics when applied to a highly inactive dialysis population. While retraining with data from dialysis patients improved the kappa statistics, classification performance remained much lower than in the healthy population. Future studies should be aware of population-specific challenges in machine-learned activity classification, and where possible collect relevant training and validation data in the disease populations of interest.
Chair
Discussant
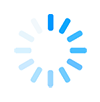