O.3.36 - Physical activity measurement and epidemiology
Saturday, June 20, 2020 |
11:45 AM - 1:00 PM |
Waihorotiu #1 Level 4 |
Details
Speaker
Capturing real-time 24-hour activity and daily weight data from Australian adults using Fitbit devices: Development of remote data collection software Fitnesslink
Abstract
Purpose: Technological advancements in consumer wearables and health technologies present exciting opportunities for remote collection of participant data for health research; however, the cost of data collection services may be prohibitive for large longitudinal studies. We aimed to develop and test software that would collect 24-hour activity and daily weight data from Fitbit devices and would enable real-time monitoring of participant compliance.
Methods: The study was registered with Fitbit to receive access to the Fitbit API and intraday time series feature. Researchers collaborated with Portal Australia to develop software, called Fitnesslink, to extract and store minute-level heart rate and activity data (categorising each minute as sedentary, light activity, moderate activity, vigorous activity or sleep according to Fitbit's proprietary algorithms) and daily weight data. To register, participants click on the provided weblink, log in using their Fitbit username and password and authorize the software to collect their data. On the researcher side, a dashboard displays all authorised devices, battery status, time of last sync, and time of last use. Alpha testing was conducted by 7 users using Fitbit Charge 3 activity trackers and Aria 2 weight scales for an average of 55 days per tester. We compared samples of extracted data with summary data shown in testers' Fitbit accounts, calculated average activity values across the full 385 days, and tested the usability of the dashboard.
Results: Extracted data exactly matched data displayed in Fitbit accounts. Data showed plausible activity values: 757 minutes sedentary; 207 minutes light; 18 minutes moderate; 20 vigorous; 438 minutes sleep per day. The dashboard provided accurate information on device status and use, but it was anticipated to be difficult to identify non-compliance among a longer list of devices. Changes were therefore made so that devices are ordered by longest time since last sync and colours are used to highlight non-compliance (e.g. if devices have not synced, or have synced but no new activity or weight data have been recorded).
Conclusions: We developed user-friendly software that collects accurate real-time Fitbit data. Fitnesslink has potential application for any research which seeks to remotely collect health data using Fitbit devices.
Criterion Validity of the Fitbit Charge 2 to Measure Physical Activity and Sedentary Behaviour in Overweight and Obese Adults
Abstract
Wearable fitness trackers have increased in popularity and could motivate individuals to live healthier lifestyles. However, the validity of wearable fitness trackers has not yet been established in an overweight population. This study aims to determine the criterion validity of the Fitbit Charge 2 in measuring physical activity (PA) and sedentary behaviour in an overweight/obese population under free-living conditions. Fifty-nine (age: M=48, SD=11 years, female: 69%) overweight/obese adults (BMI: M= 34, SD =4 kg/m2), concurrently wore a Fitbit Charge 2 tracker and an ActiGraph GT3X+ accelerometer for 8 days. Data sets were aligned so that only the same waking wear periods were included in the analysis and time spent (min/day) in sedentary time and moderate-to-vigorous physical activity (MVPA) were estimated using standard cut-points for GT3X+ and proprietary algorithms for the Charge 2. Paired t- tests and mean absolute percentage errors (MAPE) were used to examine differences and measurement errors in estimates between the two devices. Pearson and Spearman correlations and Bland-Altman (BA) plots were used to evaluate the association and potential systematic bias between the Fitbit and GT3X+. The results reflected that in comparison to the GT3X+, the Charge 2 recorded a significantly higher amount of time spent in sedentary behaviour, but had a low MAPE (mean difference [MD] = 33 min/day, p = 0.006, MAPE = 7%), whereas a relatively large MAPE was apparent for time spent in MVPA (MD = 16 min/day, p = 0.004, MAPE = 64%). The Charge 2 underestimated time spent in light PA (MD = -30 min/day, p = 0.001, MAPE = -9%) compared to the GT3X+. Despite the high degree of correlation between the two devices for step count (r=0.94, p<0.111), the Charge 2 recorded around 2000 more steps per day (p<0.001) than the GT3X+. In overweight adults, the Charge 2 overestimated time spent in sedentary behaviour, MVPA and step count compared to the GT3X+, but underestimated time spent in light PA. In this population, the Charge 2 demonstrates mostly good agreement with the GT3X+ and may be a useful tool for self-monitoring of activity.
The development and reliability of a video coding scheme for categorising free-living physical activity in 8–16 yr old children.
Abstract
Purpose: Machine learning may improve accelerometer-measured physical activity but most studies rely on laboratory data to develop ground truth measures. Studies of free-living activity are hampered by the difficulty of categorising these data. Wearable cameras can provide objective free-living ground-truth data, but there is limited literature that describes the methodological approaches used to classify the copious amount of visual data. The aim of this study is to describe the development of a coding scheme for analysing free-living physical activity video data.
Methods: GoPro videos were obtained as part of a validation of 24-hour movement behaviours in 137 children wearing wrist, hip, lower back and thigh accelerometers. Videos were imported into Noldus Observer XT. A standardized coding protocol was developed based on general movement patterns and refined through group discussion. Inter-rater reliability of second-by-second video coding was established using an iterative cycle of blind-coding (relative to other coders) followed by discussion, with all disagreements resolved by group consensus. This yielded a set of videos with criterion codes from which additional coders could be trained.
Results: Every second of video was assigned to one of 23 movement codes. Behaviour codes included lying, sitting, standing, walking, and running and whether one or both arms were engaged. Other behaviour codes included shuffling/crouching, bending, whole body physical activity, stair ascending/descending, cycling (indoor), cycling (outdoor), inactive transport (on feet), inactive transport (sitting), and swimming. Transitional movements were also coded (e.g., standing to sitting, sitting to standing, lying to standing, and standing to lying). Visual cues in the video were used to inform classification (i.e., knee, leg, and hip positions, position of hands, directional movements, and movements in relation to other objects/people in the video). Images were classified as “undefined” when the camera lens was obstructed. Two researchers independently annotated a random subsample of 30 minutes of video for each child. Inter-rater reliability was assessed using the Kappa statistic.
Conclusions: The methodological processes used in the current study can inform future studies that use wearable cameras in a free-living environment.
Temporal Physical Activity Patterns, Integrating Physical Activity Intensity and Timing, are Associated with Health
Abstract
Purpose: Patterns of physical activity (PA) frequency and intensity have been linked to obesity and chronic disease. Integration of the specific timing, intensity, and sequence of PA in a day, to create temporal PA patterns has not been attempted, despite the potential relationship with health outcomes, and is the purpose of this research.
Methods: Objectively measured PA accelerometry data from 1,627 non-pregnant US adults 20-65 years with one random valid PA day collected in the cross-sectional National Health and Nutrition Examination Survey 2003-2006 was used to pattern absolute PA intensity and time of activity using data-driven methods. Clusters representing temporal PA patterns were created using modified dynamic time warping coupled with kernel-k means clustering algorithm. Multivariate regression models controlling for potential confounders, adjusted for multiple comparisons and the complex survey design determined associations between temporal PA pattern clusters and body mass index (BMI), waist circumference (WC), fasting plasma glucose, hemoglobin A1c, triglyceride, HDL-C, total-cholesterol, systolic and diastolic blood pressure and categories obesity, type 2 diabetes, and metabolic syndrome (p<0.05/6).
Results: A cluster representing a temporal PA pattern with the highest average intensity peaking at 11:00 and tapering off throughout the day was associated with significantly lower BMI (p<0.0001), WC (p<0.0001) and 65% lower odds of obesity compared to a cluster with lower average PA intensity peaking at 12:00 and tapering off (95% CI: 0.21, 0.47) and a cluster with the lowest average PA intensity with no distinct activity peaks (95% CI: 0.17, 0.43). Another cluster representing a temporal PA pattern with high average PA intensity peaking at 19:00 was associated with significantly lower BMI (p=0.0003), WC (p=0.001) and 60% lower odds of obesity compared to a cluster with lower average PA intensity peaking at 12:00 and tapering off (95% CI: 0.20, 0.74) and a cluster with the lowest average PA intensity and no distinct activity peaks (95% CI: 0.18, 0.59).
Conclusion: Temporal PA patterns are associated with differences in U.S. adult health status indicators. This study novelly demonstrates that integrating time and intensity to temporal PA patterns differentiates health outcomes and holds promise for future interventions and potential guidelines.
Associations between parent report and accelerometer-measured physical activity and sedentary time in children: Ecological momentary assessment study
Abstract
PURPOSE: The use of mobile ecological momentary assessment (EMA) has not been investigated as a measure of young children’s physical activity (PA) and sedentary behavior (SB) when reported by a child’s parent. Our purpose was to examine the associations between parent EMA-report of child PA and SB and accelerometer-measured sedentary time (ST), light PA (LPA), and moderate-vigorous PA (MVPA), and whether these associations differed by day of week, sex, and season.
METHODS: 140 children (aged 6.4±0.8 years;47% girls) wore an accelerometer for 8 days to measure ST, LPA, and MVPA. Parent report of child PA and SB was measured via multiple daily signal contingent EMA surveys. Accelerometer data was matched to the time period occurring before parent EMA-report of child PA and SB. Generalized estimating equations with interaction-term analyses assessed whether the relationship between parent-EMA report of child PA and SB and accelerometer-measured ST, LPA and MVPA differed by day of the week, sex and season.
RESULTS: Parent EMA-report of child PA and SB was strongly associated with accelerometer-measured ST, LPA, and MVPA. Parent EMA-report of child PA was stronger during weekend than weekdays for accelerometer-measured ST (P =.001) and LPA (P=<.001). For parent EMA-report of child SB, strong associations were observed for accelerometer-measured ST (P=<.001), LPA (P=.005) and MVPA (P=.008). Sex-interaction results indicated the association between parent reported child PA via EMA and accelerometer-measured MVPA was stronger for boys than girls (P=.02). The association between parent EMA-report of child PA and SB and accelerometer-measured ST and PA was similar across seasons (all P>.1).
CONCLUSIONS: When accelerometry-based methods are not feasible in epidemiological research and in contexts where the parent is able to spend more proximate time observing the child’s PA and SB, parent EMA-report might be a superior method for measuring PA and SB in young children relative to child self-report methods -given EMA’s strong associations with accelerometer-measured PA and ST.
Re-evaluating standardised accelerometer cut-points for older adults.
Abstract
Purpose: Participation in physical activity for at least 30-minutes-a-day, and interrupting sedentary time, are recommended for older adults. Research has established standard cut-points to indicate physical activity behaviour for adults. These cut-points are typically associated with levels of activity intensity based on standardised values of resting metabolic rate (RMR). However, as older adults have lower RMR than standardised values, they need to work harder to achieve these same cut-points. Therefore, previously identified cut-points may not be applicable to older adults and lead to inaccurate assessment of time spent in sedentary, light, and moderate intensity. This research aimed to validate GENEActiv accelerometer cut-points for sedentary, light, and moderate intensity activities of daily living, against energy expenditure in older adults.
Methods: Using a test/re-test design, 34 community-dwelling older adults (70-91 years) participated in two laboratory sessions, one-week apart. During the sessions, participants completed activities of daily living, including washing dishes, light gardening, carrying shopping, unpacking shopping bags, laying down, watching television, reading a book, and sweeping. Participants wore a GENEActiv accelerometer on each wrist during the activities, with gas analysis conducted via MetaMax 3B to ascertain energy expenditure; heart rate data were also collected. Data were analysed using a specifically-designed program, Cobra Processing.
Results: Analysis identified that standard cut-points did not accurately describe the energy expenditure of older adults. Further, when worn on the non-dominant hand, the GENEActiv was not sensitive to physical activity behaviour at lower intensities. For sedentary time, both dominant and non-dominant wrist had excellent classification accuracy (sensitivity 0.99 and 0.97; specificity 0.91 and 0.86, respectively). The GENEActiv worn on the dominant wrist generally showed better accuracy.
Conclusions: Based on these findings, it is recommended that standard cut-points be modified for older adults to increase sensitivity to periods of light-to-moderate-intensity activity; and the monitor is worn on the dominant hand. Identification of cut-points that more appropriately reflect the activities of older adults will enable the accurate monitoring of sedentary, light, and moderate intensity behaviour in this population.
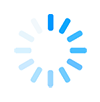