S.2.33 What can we achieve by measuring fine-grained patterns of physical activity behaviour?
Friday, June 19, 2020 |
5:00 PM - 6:15 PM |
Waihorotiu #1 Level 4 |
Details
Speaker
Using WalkScore to explain the temporal and spatial patterns of adolescent mobility and physical activity
Abstract
Purpose: To expand our understanding of the environmental determinants of physical activity, more fine-grained measures of individual behaviour are needed. Advances in measurement and data processing methodology enable the exploration of detailed temporal and spatial patterns of physical activity behaviour. This study examined how the isolation of specific activity behaviours in time and space were associated with an objective measure of neighbourhood walkability (WalkScore).
Methods: 200 adolescents (12–18 years old) wore a portable GPS receiver and an accelerometer for one week. The data were merged using the Human Activity Behavior Identification Tool and data Unification System (HABITUS). Each 15-second epoch of data were classified into one of four domains: home, school, transportation, or leisure, using the ‘palmsplusr’ R package. Activity in the transportation domain was further categorised into walking, cycling, or vehicle travel. WalkScore was calculated from each participant’s address. The association between WalkScore and (1) domain-specific activity measures (time spent in a vehicle, and distance walked) and (2) non-specific activity measures (total moderate-vigorous physical activity) was estimated using local polynomial regression.
Results: The highest proportion of time was spent at home (40.1%), followed by school (32.2%), leisure (16.9%) and transportation (9.9%). However, the highest proportion of moderate-vigorous physical activity was observed in the transportation domain (40.7%), followed by school (23.5%), home (18.5%) and leisure (16.1%). A stronger association was observed between WalkScore and distance travelled in a vehicle (R² = 0.68) and distance walked (R² = 0.34), compared to total moderate-vigorous physical activity (R² = 0.16).
Conclusions: Measuring the temporal and spatial patterns of behaviour enabled the creation of context-specific variables. Although our regression models are not directly comparable, our results suggest that WalkScore was able to explain domain-specific physical activity measures (i.e. transportation behaviour) better than non-domain-specific measures (total moderate-vigorous activity). These techniques could be used to obtain a more fine-grained understanding of the environmental determinants of health.
How do patterns of movement behaviour vary among distinct work groups?
Abstract
Purpose: Improved sensitivity to detect activity type and the transitions between them will extend our understanding of the physical demands of various occupations. This will support appropriate and tailored workplace health promotion strategies. Using a novel two-accelerometer methodology, this study examined how patterns of behaviour (e.g. sitting, standing) and their transitions (e.g. sit-to-stand) varied across distinct work groups during their working hours.
Methods: Adult employees (n = 100) from four diverse work groups in the New Zealand aviation industry (25 in each of: Office workers, Cabin crew, Airport front house, Airport back house) wore two Axivity AX3 accelerometers on their thigh and lower back for seven days. Activity type and the transitions between them were estimated using published machine learning methods and then normalised to total working hours. The activity profiles of each work group were compared using the Kruskal Wallis method with Nemenyi post-hoc pairwise comparisons.
Results: Office workers had significantly more sitting minutes during working hours (47.1 min/h) compared to the other three work groups (mean 27.3 min/h, p < 0.001). Cabin crew performed the most standing (28.7 min/h) and sit-to-stand transitions (4.5 times/h) compared to the other groups (all p < 0.05). Interestingly, front house and back house workers had frequent stand-to-walk transitions (21 and 22/h, respectively), significantly more than office workers (7.3/h) and cabin crew (14.3/h); all p < 0.05. Back house workers spent the most time walking (9.4 min/h) and had more sit‑walk transitions (4.0/h) compared to the other work groups (mean = 1.1/h); all p < 0.05.
Conclusions: These results show distinct patterns of behaviour across different work groups, indicative of the demands associated with each job type. We also observed that patterns of transition could vary between groups irrespective of the total time spent in each behaviour. This may indicate that transitions are an important consideration when understanding patterns of behaviour. The next step is to investigate how these patterns are related to health, productivity, and wellbeing.
Accessibility to public transport and patterns of walking among older adults: Combining accelerometer, GPS, and travel survey data
Abstract
Purpose: When other transport modes become hard to use for older adults, public transport can be an important asset for them to stay independent and socially active. Public transport can also be an important source of physical activity. Among people that are no longer active in the work force, walking to and from public transport hubs can form a large portion of their daily physical activity. The aim of this study is to analyse the relationship between accessibility and use of public transport among older adults (65+) in the city of Ghent, Belgium.
Methods: Travel survey data is available at a trip stage level (unimodal parts of a trip) for 850 older adults residing in Ghent. Physical activity indicators (e.g. MVPA) linked to this travel behaviour will be predicted using a random forest model based on GPS and accelerometer data from people living in Ghent (n=75). Public transport accessibility will be measured using OpenStreetMap data (e.g. distance to closest public transport hub). The associations between physical activity and public transport accessibility will be assessed with generalized additive mixed models.
Results: Preliminary results show the following transport mode shifts between 2015 and 2018 among the general population: a decrease in walking trips (15% to 13%), an increase in bicycle trips (30% to 35%), an increase in public transport trips (5% to 9%) and a decrease in private motorized trips (40% to 33%). We also expect an increase in public transport use among older adults. To unravel more precise information on the health implications of these mode shifts, physical activity indicators (e.g. daily MVPA due to transport) will be used instead of mode share. Finally, we expect that the health benefits are more pronounced among older adults living in areas with better accessibility to public transport.
Conclusions: Assessing precise patterns of mobility among older adults will allow us to quantify the effect of transport accessibility on physical activity. More broadly, it will help explain the spatial disparities in public transport use in the city. This could have important implications for future transport policies in the city of Ghent.
Chair
Discussant
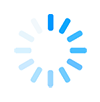