O.1.01: Charting New Territories: Cutting-Edge Methods and Measurement Advances for Enhancing Child and Family Well-being
Tracks
ISBNPA 2024 Agenda
G. Children and families (SIG)
Tuesday, May 21, 2024 |
12:00 PM - 1:15 PM |
Ballroom B |
Speaker
Ms. Olivia Finnegan
Doctoral Student
University Of South Carolina
From cybersecurity to screentime: A scoping review on motion sensor authentication and the potential in screentime measurement
Abstract
Background: Current objective measures of screentime (e.g., passive sensing applications) are unable to identify the user of a mobile device, a critical limitation in children’s screentime research where devices are often shared across a family. The field of behavioral biometrics has harnessed motion sensors embedded on mobile devices (e.g., accelerometer, gyroscope) to continuously authenticate users, yet this approach has not been applied to public health. The purpose of this scoping review was to summarize the literature on motion sensor authentication to inform the possibility of using this technology to objectively measure children’s screentime.
Methods: We systematically searched five databases (Web of Science Core Collection, Inspec in Engineering Village, Applied Science & Technology Source, IEEE Xplore, PubMed), with the last search in September of 2022. Eligible studies were published between 2007 and 2022 and included authentication of the user or the detection of demographic characteristics (age, gender) using built-in motion sensors (e.g., accelerometer, gyroscope) on mobile devices (e.g., smartphone, tablet). We extracted study characteristics (sample size, age, gender), data collection methods, data stream, and model evaluation metrics, and additionally performed a study quality assessment. Summary characteristics were tabulated and compiled in Excel. We synthesized the extracted information using a narrative approach.
Results: Of the 14,176 articles screened, 60 were included in this scoping review. The most frequently used motion sensors were accelerometer (n=59 studies) and gyroscope (n=40 studies). Most studies used an Android operating system (n=43) and were conducted in a laboratory (n=46), with specific device interaction tasks (n=49). Most studies detected a single unique user of the device (n=57). Of the studies that did report age (n=22), the majority were performed exclusively in adult populations (n=16). Study quality was low in many of the included studies, with an average score of 5.4/14.
Conclusions: Motion sensors have previously been used for user authentication in behavioral biometrics, but the existing literature is limited by low overall study quality, largely driven by lack of reporting. Freely accessible sensors, like accelerometers and gyroscopes, can potentially be used to identify children’s device use, but more rigorous research must be conducted, especially among children.
Methods: We systematically searched five databases (Web of Science Core Collection, Inspec in Engineering Village, Applied Science & Technology Source, IEEE Xplore, PubMed), with the last search in September of 2022. Eligible studies were published between 2007 and 2022 and included authentication of the user or the detection of demographic characteristics (age, gender) using built-in motion sensors (e.g., accelerometer, gyroscope) on mobile devices (e.g., smartphone, tablet). We extracted study characteristics (sample size, age, gender), data collection methods, data stream, and model evaluation metrics, and additionally performed a study quality assessment. Summary characteristics were tabulated and compiled in Excel. We synthesized the extracted information using a narrative approach.
Results: Of the 14,176 articles screened, 60 were included in this scoping review. The most frequently used motion sensors were accelerometer (n=59 studies) and gyroscope (n=40 studies). Most studies used an Android operating system (n=43) and were conducted in a laboratory (n=46), with specific device interaction tasks (n=49). Most studies detected a single unique user of the device (n=57). Of the studies that did report age (n=22), the majority were performed exclusively in adult populations (n=16). Study quality was low in many of the included studies, with an average score of 5.4/14.
Conclusions: Motion sensors have previously been used for user authentication in behavioral biometrics, but the existing literature is limited by low overall study quality, largely driven by lack of reporting. Freely accessible sensors, like accelerometers and gyroscopes, can potentially be used to identify children’s device use, but more rigorous research must be conducted, especially among children.
Biography
Olivia Finnegan, MS, is a doctoral student in the Arnold Childhood Obesity Initiative at the University of South Carolina. Olivia’s work centers around the behavioral determinants of childhood obesity (e.g., sleep, physical activity, screen time) and using technology to advance the measurement of these behaviors.
Mr. Tomisin Mayaki
Graduate Research Assistant
South Dakota State University
A methodology for assessing the validity and reliability of a national Food and Physical Activity Questionnaire for Adolescents from low-income communities
Abstract
Purpose
To further assess the validity and reliability of a 13-item Food and Physical Activity Questionnaire for Adolescents from low-income communities (FPAQ-A) used to evaluate the U.S. Expanded Food and Nutrition Education Program.
Methods
Two convenience samples of 200 low-income adolescents (grades 6-12), one for each study phase, will be recruited from four U.S. geographical regions by leveraging partnerships with Cooperative Extension. Phase One will be a reliability assessment utilizing a test-retest administration of the FPAQ-A taken 7-10 days apart. Spearman correlations will be used to assess temporal reliability and goodness of fit tests will be used to confirm the exploratory factor analysis previously conducted on the FPAQ-A (Bastian dissertation, 2022). Phase Two will assess the criterion validity of the FPAQ-A’s dietary and physical activity scales against “gold standard” measurements, i.e., dietary recall and accelerometry. The Automated Self-Administered 24-hour dietary assessment tool (ASA-24) will be used to collect diet recalls from two weekdays and one weekend day. A tri-axial accelerometer (ActiGraph GT3X-BTr) will be worn on the participants’ right hips for seven consecutive days while they maintain their normal activities. Correlation and regression analyses will be used to establish relationships between mean nutrient intake, Healthy Eating Index 2020 scores, and dietary intake questions on the FPAQ-A. Additionally, the relationship between participants' moderate-to-vigorous physical activity scores and the Physical activity questions on the FPAQ-A will be determined.
Results/findings
This methodology will be used to obtain the FPAQ-A’s temporal reliability and criterion validity estimates and confirm the previously identified factor structure. Moreover, this study will improve the external validity of the FPAQ-A, which was initially tested only with low-income adolescents in one state. Findings will be used to inform changes to the survey items if necessary.
Conclusions
This research will help improve the rigor of a nationally implemented dietary and physical activity behavior instrument that is used by other community-based nutrition education interventions across the world.
To further assess the validity and reliability of a 13-item Food and Physical Activity Questionnaire for Adolescents from low-income communities (FPAQ-A) used to evaluate the U.S. Expanded Food and Nutrition Education Program.
Methods
Two convenience samples of 200 low-income adolescents (grades 6-12), one for each study phase, will be recruited from four U.S. geographical regions by leveraging partnerships with Cooperative Extension. Phase One will be a reliability assessment utilizing a test-retest administration of the FPAQ-A taken 7-10 days apart. Spearman correlations will be used to assess temporal reliability and goodness of fit tests will be used to confirm the exploratory factor analysis previously conducted on the FPAQ-A (Bastian dissertation, 2022). Phase Two will assess the criterion validity of the FPAQ-A’s dietary and physical activity scales against “gold standard” measurements, i.e., dietary recall and accelerometry. The Automated Self-Administered 24-hour dietary assessment tool (ASA-24) will be used to collect diet recalls from two weekdays and one weekend day. A tri-axial accelerometer (ActiGraph GT3X-BTr) will be worn on the participants’ right hips for seven consecutive days while they maintain their normal activities. Correlation and regression analyses will be used to establish relationships between mean nutrient intake, Healthy Eating Index 2020 scores, and dietary intake questions on the FPAQ-A. Additionally, the relationship between participants' moderate-to-vigorous physical activity scores and the Physical activity questions on the FPAQ-A will be determined.
Results/findings
This methodology will be used to obtain the FPAQ-A’s temporal reliability and criterion validity estimates and confirm the previously identified factor structure. Moreover, this study will improve the external validity of the FPAQ-A, which was initially tested only with low-income adolescents in one state. Findings will be used to inform changes to the survey items if necessary.
Conclusions
This research will help improve the rigor of a nationally implemented dietary and physical activity behavior instrument that is used by other community-based nutrition education interventions across the world.
Biography
Tomisin Mayaki is a Graduate Research Assistant and Ph.D. student in Nutrition and Exercise Science at South Dakota State University.
Dr. Aliye Cepni
Postdoctoral Fellow
University Of South Carolina
Evaluating the performance of open-source processing of actigraphy sleep estimation in children with sleep disruptions: A comparison with polysomnography
Abstract
Purpose: Accelerometry is widely used for measuring children’s free-living sleep. The GENEActiv and GENEA data in R (GGIR) is an open-source validated method for processing 24-hour accelerometry data among adults, including sleep. However, no studies have evaluated GGIR’s performance to classify children’s sleep. This study aims to evaluate the performance of GGIR’s HDCZA sleep algorithm (van Hees et al., 2018) applied to wrist-worn accelerometry (ActiGraph GT9X Link) against polysomnography in children.
Methods: Accelerometry and polysomnography were concurrently measured in 64 children (8.6±2.3yrs, 53% female, 47% Black, 81% diagnosed sleep disorder) over a single-night lab visit. Actigraph devices were initialized to collect data at 50 Hz with idle sleep mode off. The HDCZA algorithm was applied in the GGIR (2.10.3) to code 30-second epochs as sleep and wake. The GGIR's basic sleep log function with time in and out of bed noted by the sleep techs was used. Standard scoring was applied for polysomnography data. Epoch-by-epoch and discrepancy analyses for sleep metrics [wake after sleep onset (WASO), total sleep time (TST), sleep efficiency (SE), and sleep onset latency (SOL)] determined the level of agreement between accelerometry and polysomnography.
Results: Sleep-wake estimation accuracy using accelerometry compared to polysomnography was 72.3%±10.2, with sensitivity for sleep detection at 73.9%±10.7 and wake detection specificity at 55.2%±28.2. Mean bias values were for WASO (56.6 min), TST (-78.0 min), SE (-17.7%), and SOL (21.4 min). Positive values indicate overestimation, negatives suggest underestimation compared to polysomnography. Mean absolute errors were 58.4 minutes (WASO), 79.6 minutes (TST), 18.1% (SE), and 35.2 minutes (SOL). All sleep measures, except WASO, showed a negative proportional bias. Accelerometry consistently underestimated SE as polysomnography-derived SE increased. Accelerometry overestimated TST and SOL for participants with lower polysomnography-derived values and underestimated those with higher polysomnography-derived TST and SOL.
Conclusions: Like other common algorithms (Sadeh and Cole-Kripke) on Actigraph data of children, the HDCZA sleep algorithm was more sensitive to detecting sleep but less specific to detecting wake. The algorithm performed poorly in estimating sleep metrics. These findings may be limited to children with diagnosed sleep disorders. The algorithm needs further testing in children without sleep disorders.
Methods: Accelerometry and polysomnography were concurrently measured in 64 children (8.6±2.3yrs, 53% female, 47% Black, 81% diagnosed sleep disorder) over a single-night lab visit. Actigraph devices were initialized to collect data at 50 Hz with idle sleep mode off. The HDCZA algorithm was applied in the GGIR (2.10.3) to code 30-second epochs as sleep and wake. The GGIR's basic sleep log function with time in and out of bed noted by the sleep techs was used. Standard scoring was applied for polysomnography data. Epoch-by-epoch and discrepancy analyses for sleep metrics [wake after sleep onset (WASO), total sleep time (TST), sleep efficiency (SE), and sleep onset latency (SOL)] determined the level of agreement between accelerometry and polysomnography.
Results: Sleep-wake estimation accuracy using accelerometry compared to polysomnography was 72.3%±10.2, with sensitivity for sleep detection at 73.9%±10.7 and wake detection specificity at 55.2%±28.2. Mean bias values were for WASO (56.6 min), TST (-78.0 min), SE (-17.7%), and SOL (21.4 min). Positive values indicate overestimation, negatives suggest underestimation compared to polysomnography. Mean absolute errors were 58.4 minutes (WASO), 79.6 minutes (TST), 18.1% (SE), and 35.2 minutes (SOL). All sleep measures, except WASO, showed a negative proportional bias. Accelerometry consistently underestimated SE as polysomnography-derived SE increased. Accelerometry overestimated TST and SOL for participants with lower polysomnography-derived values and underestimated those with higher polysomnography-derived TST and SOL.
Conclusions: Like other common algorithms (Sadeh and Cole-Kripke) on Actigraph data of children, the HDCZA sleep algorithm was more sensitive to detecting sleep but less specific to detecting wake. The algorithm performed poorly in estimating sleep metrics. These findings may be limited to children with diagnosed sleep disorders. The algorithm needs further testing in children without sleep disorders.
Biography
Dr. Cepni's research aims to improve children's eating and activity behaviors to address health disparities. Dr. Cepni received her doctorate degree in Kinesiology from the University of Houston, where she studied the impact of school-based physical activity programs on child executive functioning and obesity-related health outcomes. She is currently working on her K99/R00 grant proposal to prevent obesogenic behaviors among adolescents that lead to BMI gain over the summer.
Dr. Robert Weaver
Associate Professor
University Of South Carolina
Jerks are useful: Extracting pulse rate from wrist-placed accelerometry jerk during sleep in children.
Abstract
Purpose: This study estimated children’s pulse-rate via wrist placed accelerometry and compare these estimates to electrocardiogram (ECG) as a gold standard.
Methods: Children (N=82, M=8.4yrs SD=2.2yrs, 51% male) wore a consumer wearable (Apple Watch Series 7) and a wrist-placed research grade accelerometer (ActiGraph GT9X) while undergoing an overnight laboratory-based polysomnography, including a 3-lead ECG. Raw accelerometry data from Apple was extracted using SensorLog, a freely available user-written application that uses the devices’ application programming interface. ActiGraph raw accelerometry data was extracted via Actilife Software. All subsequent processing was performed in MATLAB. Pulse-rate estimates from the raw accelerometry data were calculated from peak magnitude frequency in short time Fourier Transforms of Hilbert transformed jerk computed from acceleration magnitude. Heartrates from the criterion ECG were estimated from R-R spacings using R-pulse detection in normalized ECG traces. Agreement of accelerometer derived pulse rate with ECG was assessed via Pearson Correlation (r), Lin’s Concordance Correlation Coefficient (LCCC), Mean absolute error (MAE), and mean absolute percent error (MAPE) overall and within each sleep stage.
Results/findings: For ActiGraph, r and LCCC were weak at -0.12 and -0.11, respectively, while MAE and MAPE were high at 16.8 (SD=14.2) beats/minute and 20.4% (SD=18.5%). For Apple r, and LCCC were moderate at 0.65 and 0.61, respectively, while MAE and MAPE were 6.4 (SD=9.9) beats/minute and 7.3% (SD=10.3%). For Apple, there was stronger agreement between accelerometer estimate pulse-rate and ECG estimated heartrate in stage N2 (r=0.78, LCCC=0.77, MAE=4.5 SD=6.8, MAPE=5.7% SD=8.4%), N3 (r=0.81, LCCC=0.80, MAE=4.0 SD=6.7, MAPE=4.8% SD=7.8%), and REM (r=0.78, LCCC=0.76, MAE=5.0 SD=6.6, MAPE=5.8% SD=7.2%) than during sleep stage N1 (r=0.47, LCCC=0.38, MAE=13.4 SD=13.9, MAPE=15.4% SD=20.4%) or time awake (r=0.33, LCCC=0.22, MAE=14.6 SD=14.1, MAPE=15.0% SD=12.7%) when children were likely moving more. AcitGraph’s performance was equally poor across sleep stages.
Conclusions: Raw accelerometry data extracted from Apple but not ActiGraph could be used to estimate pulse-rate in children while they sleep. Additional work is needed to explore the sources (i.e., hardware, software, etc.) of discrepancy for ActiGraph, as accelerometer estimated pulse-rate could be used as a physiological signal in sleep detection and to determine wear-time vs. non-wear time.
Methods: Children (N=82, M=8.4yrs SD=2.2yrs, 51% male) wore a consumer wearable (Apple Watch Series 7) and a wrist-placed research grade accelerometer (ActiGraph GT9X) while undergoing an overnight laboratory-based polysomnography, including a 3-lead ECG. Raw accelerometry data from Apple was extracted using SensorLog, a freely available user-written application that uses the devices’ application programming interface. ActiGraph raw accelerometry data was extracted via Actilife Software. All subsequent processing was performed in MATLAB. Pulse-rate estimates from the raw accelerometry data were calculated from peak magnitude frequency in short time Fourier Transforms of Hilbert transformed jerk computed from acceleration magnitude. Heartrates from the criterion ECG were estimated from R-R spacings using R-pulse detection in normalized ECG traces. Agreement of accelerometer derived pulse rate with ECG was assessed via Pearson Correlation (r), Lin’s Concordance Correlation Coefficient (LCCC), Mean absolute error (MAE), and mean absolute percent error (MAPE) overall and within each sleep stage.
Results/findings: For ActiGraph, r and LCCC were weak at -0.12 and -0.11, respectively, while MAE and MAPE were high at 16.8 (SD=14.2) beats/minute and 20.4% (SD=18.5%). For Apple r, and LCCC were moderate at 0.65 and 0.61, respectively, while MAE and MAPE were 6.4 (SD=9.9) beats/minute and 7.3% (SD=10.3%). For Apple, there was stronger agreement between accelerometer estimate pulse-rate and ECG estimated heartrate in stage N2 (r=0.78, LCCC=0.77, MAE=4.5 SD=6.8, MAPE=5.7% SD=8.4%), N3 (r=0.81, LCCC=0.80, MAE=4.0 SD=6.7, MAPE=4.8% SD=7.8%), and REM (r=0.78, LCCC=0.76, MAE=5.0 SD=6.6, MAPE=5.8% SD=7.2%) than during sleep stage N1 (r=0.47, LCCC=0.38, MAE=13.4 SD=13.9, MAPE=15.4% SD=20.4%) or time awake (r=0.33, LCCC=0.22, MAE=14.6 SD=14.1, MAPE=15.0% SD=12.7%) when children were likely moving more. AcitGraph’s performance was equally poor across sleep stages.
Conclusions: Raw accelerometry data extracted from Apple but not ActiGraph could be used to estimate pulse-rate in children while they sleep. Additional work is needed to explore the sources (i.e., hardware, software, etc.) of discrepancy for ActiGraph, as accelerometer estimated pulse-rate could be used as a physiological signal in sleep detection and to determine wear-time vs. non-wear time.
Biography
My work focuses on helping professionals that teach and care for school age children to create safe and healthy environments. I am currently conducting research in schools and out of school time programs to address unhealthy weight gain in youth. I have expertise in physical education, promotion of youth healthy eating and physical activity, and measurement of healthy eating and physical activity.
Dr. Teresia O'Connor
Professor of Pediatrics
Baylor College of Medicine, Department of Pediatrics, USDA/ARS Children's Nutrition Research Center
FLASH-TV 3.0: Validation of FLASH-TV methods for estimating TV-viewing among children
Abstract
Purpose: TV-viewing is a sedentary behavior that may have health risks. Existing tools to measure children’s TV viewing are insufficient. Our goal was to validate a system for passively measuring the time children spend watching TV: FLASH-TV 3.0.
Methods: A task-based study (n=30) was conducted with family-triads (parent and 2 siblings) stratified by child race and ethnicity. Family-triads participated in screen use protocols for about 90-minutes in an observation lab. FLASH-TV collects video data and process it with machine learning algorithms to estimate a child’s TV viewing time by sequentially detecting faces in the video, verifying whether faces were the target child, and assessing TV-watching (gaze) of the target child. The video-data were assessed at 5-second epochs. Only the processed TV-viewing output was saved by FLASH-TV. Video data collected by a separate identical camera in close proximity to FLASH-TV, was coded by staff using duration coding for whether the target child’s gaze was on the TV = gold-standard (10% double coded, mean Kappa 0.89 (SD 0.07)). The FLASH-TV output was compared to the gold standard for sensitivity, specificity, overall TV viewing (min:sec) calculated using intra-class correlation (ICC) in a generalized linear mixed model, and epoch by epoch correlations via Kappas.
Results: The sample was comprised of 30% black, 27% Hispanic white, 27% non-Hispanic white, and 17% other target children and the mean age was 8.3 years. Epoch-by-epoch correspondence of FLASH-TV with gold standard had a mean Kappa of 0.70 (SD 0.16), sensitivity of 77.8% (SD 17.3%), and specificity of 88.4% (SD 6.5%). The ICC was 0.83 when comparing the child’s gold standard TV viewing time (minutes) to FLASH-TV’s estimated time. The ICC varied by children’s race and ethnicity, with the lowest ICC among black youth (ICC=0.62) and the highest among other youth (ICC 0.99).
Conclusion: FLASH-TV 3.0 is a valid tool to accurately measure children’s TV viewing, however there is variability according to children’s race and ethnicity. Future studies should assess if bias is introduced by the machine learning algorithms or is due to behavioral differences in watching TV among children. Further validation in children’s homes is underway.
Methods: A task-based study (n=30) was conducted with family-triads (parent and 2 siblings) stratified by child race and ethnicity. Family-triads participated in screen use protocols for about 90-minutes in an observation lab. FLASH-TV collects video data and process it with machine learning algorithms to estimate a child’s TV viewing time by sequentially detecting faces in the video, verifying whether faces were the target child, and assessing TV-watching (gaze) of the target child. The video-data were assessed at 5-second epochs. Only the processed TV-viewing output was saved by FLASH-TV. Video data collected by a separate identical camera in close proximity to FLASH-TV, was coded by staff using duration coding for whether the target child’s gaze was on the TV = gold-standard (10% double coded, mean Kappa 0.89 (SD 0.07)). The FLASH-TV output was compared to the gold standard for sensitivity, specificity, overall TV viewing (min:sec) calculated using intra-class correlation (ICC) in a generalized linear mixed model, and epoch by epoch correlations via Kappas.
Results: The sample was comprised of 30% black, 27% Hispanic white, 27% non-Hispanic white, and 17% other target children and the mean age was 8.3 years. Epoch-by-epoch correspondence of FLASH-TV with gold standard had a mean Kappa of 0.70 (SD 0.16), sensitivity of 77.8% (SD 17.3%), and specificity of 88.4% (SD 6.5%). The ICC was 0.83 when comparing the child’s gold standard TV viewing time (minutes) to FLASH-TV’s estimated time. The ICC varied by children’s race and ethnicity, with the lowest ICC among black youth (ICC=0.62) and the highest among other youth (ICC 0.99).
Conclusion: FLASH-TV 3.0 is a valid tool to accurately measure children’s TV viewing, however there is variability according to children’s race and ethnicity. Future studies should assess if bias is introduced by the machine learning algorithms or is due to behavioral differences in watching TV among children. Further validation in children’s homes is underway.
Biography
Teresia O’Connor is a practicing general pediatrician and fellowship trained clinician researcher. Her research focuses on
a) Identifying strategies to best prevent and treat childhood obesity.
b) Providing better understanding of how parents (fathers and mothers) influence their children’s obesity-related behaviors through the framework of parenting styles and parenting practices.
c) Assessing how to use technology to improve the measurement of children’s obesity-related behaviors.
Mr. Zakaria Doueiri
Research Assistant, Implementation Coordinator
Stanford University School of Medicine
Enhancing Effective Dialogue with Community Decision-makers around Caregiver Health in Bogotá, Colombia Using Virtual Reality
Abstract
Enhancing Effective Dialogue with Community Decision-makers around Caregiver Health in Bogotá, Colombia Using Virtual Reality
Zakaria N. Doueiri (corresponding author, presenting author), Paula Guevara-Aladino (corresponding author), Olga L. Sarmiento (corresponding author), María Alejandra Rubio, Lina María Gómez-García, Diego Martínez, Abby C. King (corresponding author), Adriana Hurtado, Ann Banchoff, Luis A. Guzmán, María José Álvarez, Leonardo Palencia
Introduction: Nearly 90% of women engage in unpaid care work in Bogotá, Colombia. Unpaid caregivers experience increased rates of physical inactivity and sedentary behavior, which put them at heightened risk for stress-related health conditions, including increased depression and reduced quality of life. The Manzanas del Cuidado (Care Block) program provides health-enhancing opportunities to address health inequities among caregivers. Study aims were to i) characterize the experiences of unpaid caregivers regarding physical activity (PA) and well-being; ii) identify caregivers’ perceived built and social environment facilitators and barriers to access and use of the Care Block facility; and iii) document a community-led data generation and advocacy process using virtual reality to further advance support for the Care Block program.
Methods: The Our Voice method fosters resident participation and intersectoral collaboration for change using the Discovery Tool (DT) mobile application. Participants used the DT to identify facilitators and barriers when traveling to the Care Block. Based on data “hotspots” from caregivers’ walk audits, a virtual reality (VR) experience was developed for local policymakers and other stakeholders to visualize the caregivers’ experiences. Four community meetings were then held where participants collaboratively categorized their photos into key themes, prioritized solutions, and presented them to decision-makers.
Results: Participants (N=21) collected 257 photographs+audio comments addressing what makes it easy or hard to visit the Care Block program. Of these photos+narratives, 67% (N=172) identified negative features, 28% (N=73) positive features, and 5% (N=12) neutral features. Caregivers highlighted the presence of Care Block PA services/facilities (N=37; 39%) and transportation (N=15; 19%) as the main facilitators of PA and well-being. Poor quality/lack of sidewalks and roads (N=64; 36%), insecurity (N=41; 24%), and high risk of pedestrian-vehicle collisions (N=17; 10%) were identified as the main barriers to PA. VR-collected information was rated as easy to use (67% agreed), helped to promote compelling dialogue (71% agreed) and advanced innovative solution-building (81% agreed) among participants and decision makers.
Conclusion: VR+Our Voice enhanced positive interactions and understanding among unpaid caregivers and community stakeholders, fostering collective reflection about an urban initiative that facilitates the equitable distribution and support of care work.
Zakaria N. Doueiri (corresponding author, presenting author), Paula Guevara-Aladino (corresponding author), Olga L. Sarmiento (corresponding author), María Alejandra Rubio, Lina María Gómez-García, Diego Martínez, Abby C. King (corresponding author), Adriana Hurtado, Ann Banchoff, Luis A. Guzmán, María José Álvarez, Leonardo Palencia
Introduction: Nearly 90% of women engage in unpaid care work in Bogotá, Colombia. Unpaid caregivers experience increased rates of physical inactivity and sedentary behavior, which put them at heightened risk for stress-related health conditions, including increased depression and reduced quality of life. The Manzanas del Cuidado (Care Block) program provides health-enhancing opportunities to address health inequities among caregivers. Study aims were to i) characterize the experiences of unpaid caregivers regarding physical activity (PA) and well-being; ii) identify caregivers’ perceived built and social environment facilitators and barriers to access and use of the Care Block facility; and iii) document a community-led data generation and advocacy process using virtual reality to further advance support for the Care Block program.
Methods: The Our Voice method fosters resident participation and intersectoral collaboration for change using the Discovery Tool (DT) mobile application. Participants used the DT to identify facilitators and barriers when traveling to the Care Block. Based on data “hotspots” from caregivers’ walk audits, a virtual reality (VR) experience was developed for local policymakers and other stakeholders to visualize the caregivers’ experiences. Four community meetings were then held where participants collaboratively categorized their photos into key themes, prioritized solutions, and presented them to decision-makers.
Results: Participants (N=21) collected 257 photographs+audio comments addressing what makes it easy or hard to visit the Care Block program. Of these photos+narratives, 67% (N=172) identified negative features, 28% (N=73) positive features, and 5% (N=12) neutral features. Caregivers highlighted the presence of Care Block PA services/facilities (N=37; 39%) and transportation (N=15; 19%) as the main facilitators of PA and well-being. Poor quality/lack of sidewalks and roads (N=64; 36%), insecurity (N=41; 24%), and high risk of pedestrian-vehicle collisions (N=17; 10%) were identified as the main barriers to PA. VR-collected information was rated as easy to use (67% agreed), helped to promote compelling dialogue (71% agreed) and advanced innovative solution-building (81% agreed) among participants and decision makers.
Conclusion: VR+Our Voice enhanced positive interactions and understanding among unpaid caregivers and community stakeholders, fostering collective reflection about an urban initiative that facilitates the equitable distribution and support of care work.
Biography
Zakaria Doueiri is a research assistant and implementation coordinator at the Stanford Prevention Research Center. During his undergraduate career at Stanford University, he studied the intersection of neurobiology and social determinants of health and developed an interest in learning how technology can be leveraged to offer more equitable outcomes for underserved populations. For his senior thesis project, he investigated the integration of virtual reality into the Our Voice method to better visualize and document the perspectives of unpaid care workers in Bogotá, Colombia.
Chair
Teresia O'Connor
Professor of Pediatrics
Baylor College of Medicine, Department of Pediatrics, USDA/ARS Children's Nutrition Research Center
Co-chair
Aliye Cepni
Postdoctoral Fellow
University Of South Carolina
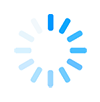